
Detection Results Overview & Side by Side image comparison to validate results.
- Availability of data. Unfortunately, up-to-date and high-quality geospatial data is not always easily available in every location in the world. That’s why drone monitoring services are crucial in data collection, and drone operators are equipped with high-resolution cameras.
- The number of resources that must be spent on analyzing the data. Investigating imagery, counting objects, and looking for patterns manually is extremely time-consuming, tedious, and prone to errors. Analyzing large locations in such a way is often impossible in a reasonable time. Machine learning-based models solve this issue, and Picterra is a perfect solution for that purpose.
Fire risk assessment with UAVs and AI
Our user, C2 Group, which is a full-service program, project, and construction management firm with a focus on technology integration, has been utilizing drones as a tool to identify fire risk since 2016 and has for the last 2 years been using AI and ML to take on the tasks of risk assessment. First, drone field teams start with collecting the data. Flight patterns and paths are derived based on the terrain and data quality objectives and then the type of UAV and payload is selected based on the specific needs of each project. C2 Group has been using the SONY A7R4 61 megapixel and A7R3 42 megapixel cameras paired with heavy lift DJI M600Pro and Intel Falcon 8+ drones for extremely detailed results. High resolution cameras paired with precision drones allow gathering enormous amounts of high-quality data that is necessary for AI-based data processing. Large scale projects can require upwards of 90,000 images that must be georeferenced and then stitched together into a single orthorectified image. This is the next step in the process. Powerful equipment is crucial here, and computers are being built specifically for handling such amounts of data. Their computing power and processing settings have to meet the data quality objectives from end-users. C2 Group has used multiple overclocked 28 core processor computers running over a terabyte of RAM. In addition, multiple Nvidia Titan graphics cards are used to handle the processing workflow and expedite the front-end data processing.
The next step is creating the AI workflow which needs to meet the project’s objectives in terms of speed, accuracy, resources, and the final cost. This is where Picterra shines. With it’s easy to use interface and advanced machine learning algorithms, Picterra can take on the most demanding distributed computing AI tasks while reducing deliverable costs.
For this AI workflow, after uploading the imagery our users build deep learning-based detectors by themselves, with no coding skills required at any stage. With Picterra, it’s possible to detect various objects that are crucial in fire risk assessment, such as buildings, multiple types of vegetation, dead tree canopies, tanks, etc.
With just a handful of annotations on your imagery, Picterra takes your work and feeds it directly to a deep learning model, training it to perform your desired task at scale. The model is automatically trained on a cloud-based GPU infrastructure and then can be applied to the rest of your imagery via said GPUs as well. Picterra’s cloud infrastructure automatically scales to the number of detection tasks that you need to run through. As a user, this happens all “underneath the hood” so to speak and you don’t have to worry about computational resource allocation or task assignments yourself.
Timewise, the results are ready in an extraordinarily short time – often in less than an hour, including annotations (in most cases, it’s enough to add a few) and training the detector. To compare, achieving similar results manually without the platform and its algorithms would require weeks of work. The final cost would be significantly higher.
Detecting buildings
Analyzing different types of buildings is usually a priority in fire risk assessment, especially in geographic conversion zones that border urban developments. In theory, it could be based on real property records, but not every region will have this information available digitally. Besides, informal settlements are also not uncommon. In light of these facts, it’s crucial to get up-to-date data and to take action based on the real situation. Picterra is an excellent example of the platform that allows for building the above mentioned AI workflows on satellite, aerial, and drone imagery. Because the platform is highly flexible and is not limited to specific use cases, Picterra’s algorithms can be trained to accurately detect very different types of buildings – American style, European style, slums, both industrial and residential buildings, refugee camps, and so on. With Picterra, it’s fast, efficient and doesn’t require coding or machine learning skills. In short, all you need to do to detect buildings is to:- Upload imagery. We facilitate accessing satellite imagery through our portal. However, for fire risk management, you probably need to bring your own up-to-date and local data.
- Train your machine learning model. We’ve covered it in detail in this step-by-step guide on how to build a custom detector, and we recommend reading it before moving on.
- Picterra is remarkably fast, but you can get even faster by using our pre-built detector, which is ready to detect buildings.
- Your results are ready. You can share them or download them to integrate with other GIS software you may use.
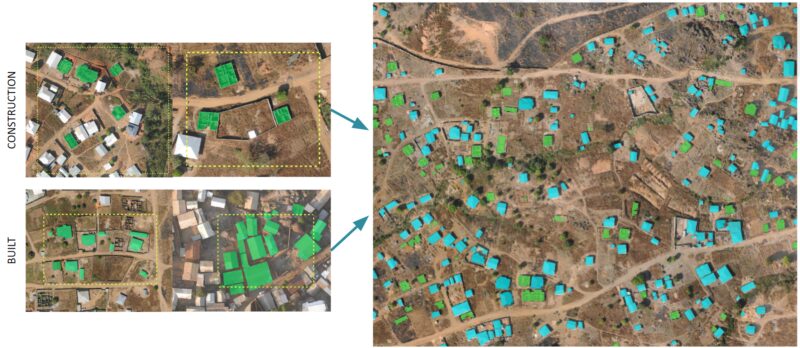
Example of buildings detector built on satellite imagery from Cameroon.
Detecting vegetation
In fire risk assessment, it can also be essential to analyze the surrounding vegetation. On Picterra, you can do it precisely the way we presented when detecting buildings. You need to import imagery, choose your training areas, add a few annotations, and build a detector. You may need multiple detectors for different vegetation classifications, but the same process will apply to detector creation. Or take a shortcut using one of our pre-trained, base detectors to test your imagery based on predetermined detection criteria.
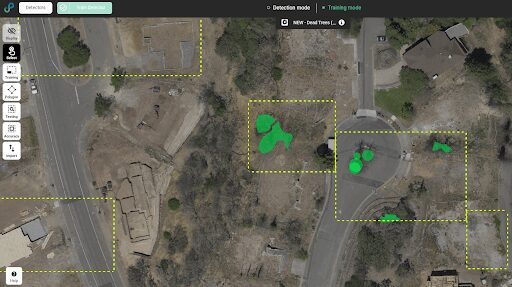
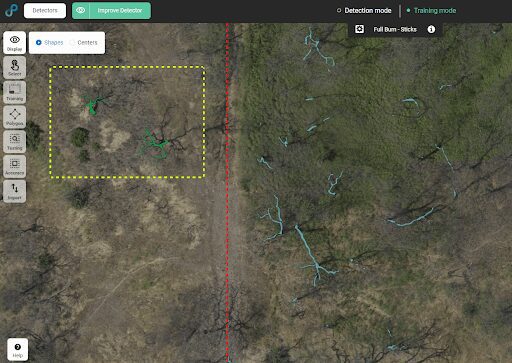
Example of Custom Detector built to identify fully burnt trees while avoiding shadows. Inclusion objects highlighted with green polygon, test detection objects highlighted in light blue.
Fire risk assessment – summary
The final piece of the puzzle is merging all of the detector data together with outside data sources to assess the potential risk areas. C2 Group uses the ESRI Shape File outputs of the detector data for use in ArcGIS. They are then combining the detector data with additional modeling features for slope analysis, weather modeling, historic images, and change detection overlays to create a full risk profile of the surveyed area. When it comes to safety, there is no room for error. Fire risk assessment requires both up-to-date data and state-of-the-art technology. Never before have both been as accessible as now – drone monitoring services are widely available; and with Picterra, everyone can build a highly accurate custom machine learning-based AI model.
Results Report – All custom detectors built for this project.
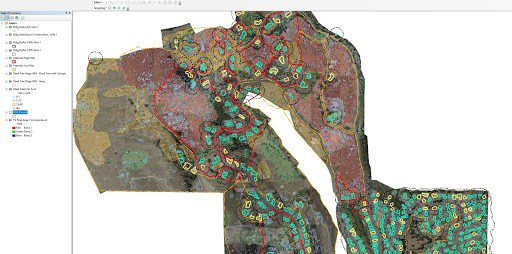
Picterra shapefile output merged with property data, slope analysis & encroachment buffers using ESRI ArcGIS.
AI in object detection – what else can be done with Picterra
It’s worth mentioning that Picterra is not limited to detecting buildings and vegetation. As the leading platform for geospatial machine learning, the platform is extremely flexible and highly evolved for use in precision agriculture, civil engineering, disaster management, master planning, safety management, and several other applications. It’s even safe to say that if you can see an object on a drone, aerial, or satellite imagery, Picterra can detect it. Picterra’s users have detected and compared the number of buildings vs. ongoing constructions, assessed road conditions, detected harmful weeds, and various different objects and patterns.
Authors:
Brandon Del Priore, Chief Technology Officer at C2 Group
Monika Ambrozowicz, Product Marketing Manager at Picterra