Picterra is a company that sits at the intersection of two rising domains. The first is the geospatial domain and more specifically Earth Observation imagery. The second is Machine Learning. Our end goal has always been to democratise the access and use of both technologies. As a part of that, we must always remember that we have to be aware of the limits and boundaries of these technologies, and then further that we have to try and push those boundaries. This post will be the first of two about some of our preliminary work into two specific topics that many would consider “cutting-edge”, relating to each of these two domains. For Earth Observation imagery we will take a look at Synthetic Aperture Radar (SAR) data. For machine learning, we’ll be summarising our investigations with Capsule Networks. Let’s start with SAR data.
What is Synthetic Aperture Radar (SAR)?
SAR imagery has been around for a while, but it has mostly only been used for military purposes. Only recently has it come to the attention of private companies as a potential way to unlock the benefits of radar data. And so what are these benefits exactly? Well it can see through those pesky clouds for one thing, and it also doesn’t matter whether it’s night or day, the resulting image will look the same (so you could say it has night vision). SAR does not use the optical (red, green, blue, etc.) wavelength as regular cameras so it captures completely different properties of objects since it interacts with those objects differently. Certainly, you could wait for a nice sunny cloud-less day over an area of interest but in many regions of the world, this is quite difficult to come by, making SAR data a great choice, especially in monitoring or alerting based applications where periodic data over a region is required.
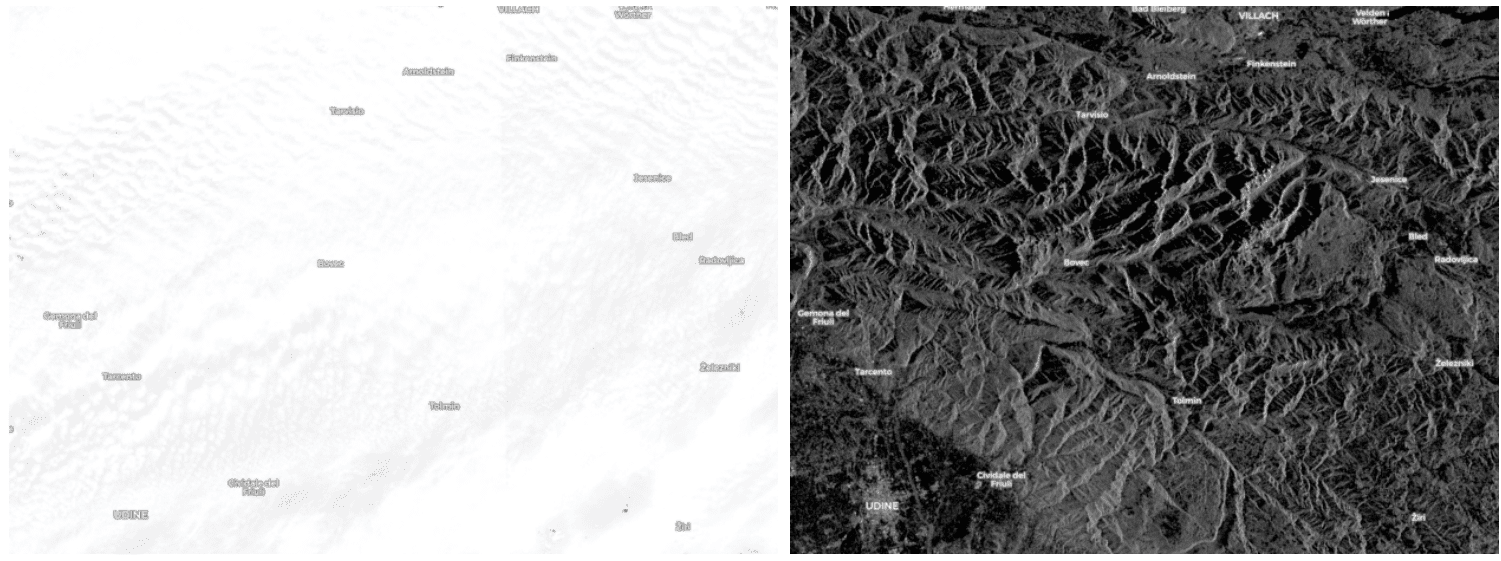
Two images over the same region (over a mountain range) and the same time, but on the left is the RGB image which is completely cloud covered, and on the right the SAR version, which sees through the clouds.
Other benefits are that it can capture features such as the roughness of surfaces. This is one reason why it is oftentimes used for maritime applications. Since the sea is usually quite uniformly rough, anything that isn’t the same “roughness” like a boat, really stands out. However, this also means that you should not think of SAR as just a night vision, a cloud-free equivalent of RGB data. You really have to choose your use case correctly. For example, if you’re trying to map building footprints (which are stationary anyways), you’re probably better off waiting for that sunny day and using RGB imagery. But if you’re trying to monitor deforestation over time in a tropical zone where there are clouds 90% of the year, then SAR may be your best bet. We’ll show you an example of building detection with SAR later but that’s only because it was the largest existing SAR dataset we could find.
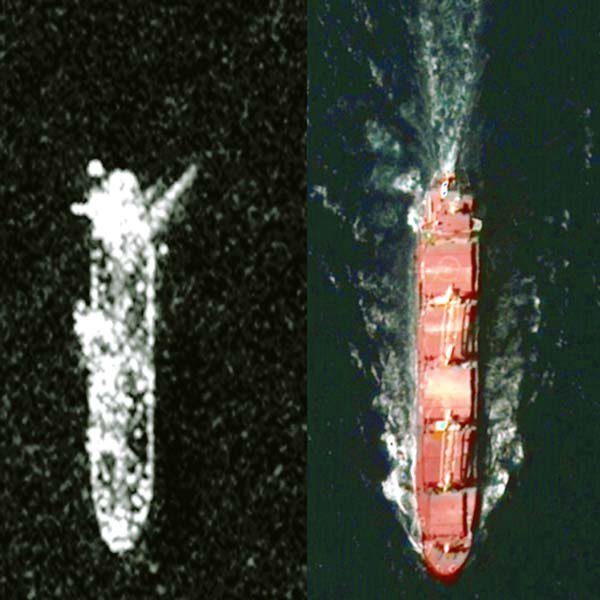
A boat in SAR (left) and RGB (right)
Some other things to be aware of while working with SAR is that images are often off-nadir and also quite noisy (as you have may have noticed in the images above) due to the physical properties of the technology. There is certainly a lot of research that goes into “cleaning up” this data, but that’s just something to be aware of if you choose to use it. In addition, there are many configurations of SAR data, different polarisations and wavelengths for example. Each configuration captures different things so it’s important to choose the right configuration for the right job, a problem that is not yet super well explored.
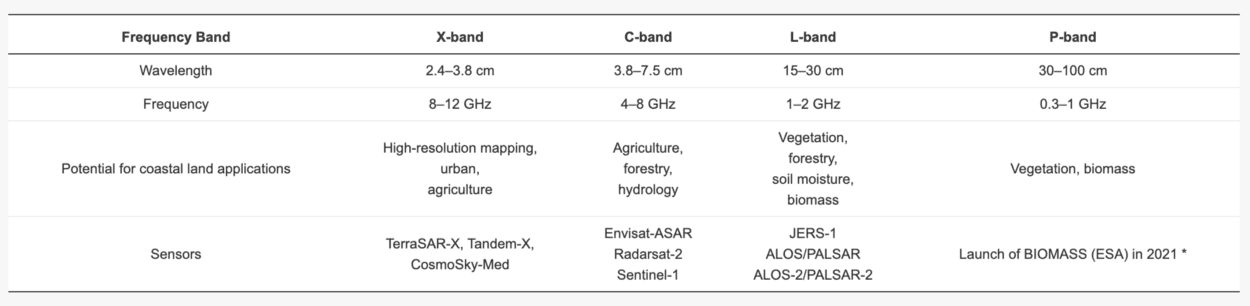
A table of different SAR band types and some of their potential application areas, taken from MDPI: https://www.mdpi.com/2072-4292/12/14/2228/htm
Additionally, it is quite difficult to come by SAR imagery. As I said, it used to be used mainly for military applications. It still is, in fact, especially the higher resolution data. The result is that it is very expensive and in some ways exclusive, but as more SAR imagery service providers start to appear, such as ICEye, Cappella Space, etc, we are optimistic that these barriers will eventually be lifted, democratising the use of this powerful technology for hopefully more positive and altruistic applications.
Experimenting with SAR data
Now on to our tests with SAR imagery. Namely, we tried to train the Spacenet 6 dataset, which is of 4 channel SAR and 3 channel RGB imagery over a port in Rotterdam, using the deep learning technology that powers the Picterra platform. The results are visualised below with our detections on the left and the ground truth on the right.
From such “fuzzy” looking data, it is quite amazing to see that we at least still manage to find the majority of the larger buildings. On the smaller buildings it seems to struggle, as the data is not as crisp as the RGB version so it’s harder to pick out finer details. Though our results with the RGB data have some trouble with these as well. It still is true that RGB does perform quite a bit better for buildings in this case, as I mentioned it would previously. Here are some results from training just on the RGB data.
You may be thinking that SAR is a poor choice now, but remember that this was just a proof of concept to show we can indeed get something out of SAR data, despite its increased complexity, which is a good indication that it can utilised for those use cases that RGB imagery would not be able to handle at all (seeing through clouds, night-time images, etc). Moving forwards the next initiative would be to increase accessibility to this data and encourage the discovery of new use cases that can really take advantage of the combination of SAR and machine learning. The next steps we need to take towards this goal are certainly less technical in nature and more about communication and outreach, but that is a necessary and significant task in itself.
Working with SAR data may seem daunting at first, but with some effort and persistence, we’re hopeful that we can make it increasingly easy to obtain and work with this data, unlocking the power of SAR for all!
Read our next post, where we’ll be switching attention to pushing the limits with machine learning and in particular with something called Capsule Networks.
Picterra out!