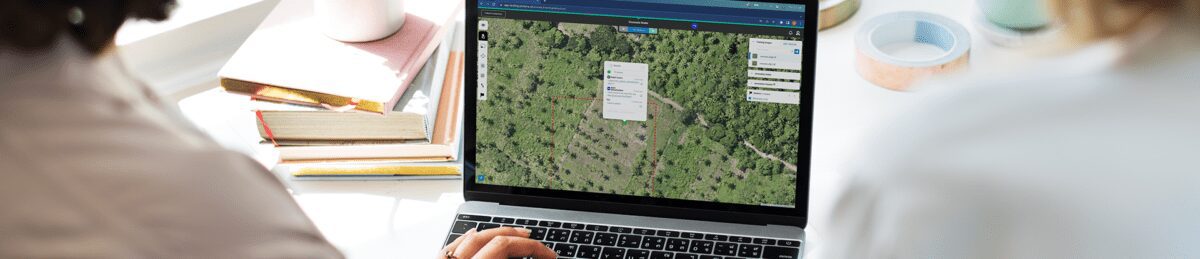
Customer stories
How Cyient saves time & effort by using MLOps and Picterra to enable their geospatial practice
Industry:
Agriculture
Business type:
Leading global engineering and technology solutions

Overview
An often quoted statistic and widely cited article from VentureBeat states that only 13% of data science projects ever make it into production. Given that this statistic is already a few years old does it still hold true and what recent advancements have been made in the world of machine learning (ML) to ensure this number increases?
We recently hosted a webinar with Cyient, a leading global engineering and technology solutions company, to delve into this question and discover how MLOps and Picterra enable their geospatial practice. Read on to learn more and how it can be applied within your organization to help you scale and prototype faster.

What is geospatial MLOps?
In recent years ML has become much more applied and less theoretical with businesses across industry beginning to incorporate it into strategic projects. As a result, data scientists are becoming more visible by their ability to tie their work to business impact. However the struggle lies in productionizing their work due to resource constraints. This could be because of perceived risk and lack of certainty causing their work to lose priority. Therefore, greater alignment between business, IT, and data science teams is crucial to re-address this balance and ensure that data science teams are not siloed or separated.
Enter MLOps. This term may sound familiar for those who have worked on traditional software projects, because it is closely tied to DevOps, the “parent” of MLOps. MLOps is a set of practices that aims to deploy and maintain machine learning models in production reliably and efficiently. When an algorithm is ready to be launched, MLOps is practiced between data scientists, DevOps, and machine learning engineers to transition the algorithm to production systems.
Commercial imaging companies are collecting upwards of 200 terabytes of earth observation (EO) data every day, 365 days a year, accumulating huge data sets measured in petabytes per company. Yet there is a bottleneck for being able to put this data to use for business. Often it requires a GIS specialist to collaborate with data scientists – and there are not enough of those to go around. Geospatial MLOps makes machine learning projects available for new companies that in the past wouldn’t have had the available budget or work force. A scalable software platform like Picterra allows companies to build applications and services to detect objects, monitor changes, and spot patterns in satellite, drone, and aerial imagery.

Picterra provides a full software platform for geospatial ML – all the way from data processing to integrating with third-party reporting software.
How geospatial MLOps supports business expansion
During the webinar we were joined by Earth Observation Consultant Dr. Yajnaseni Palchowdhuri and she shared her perspective on the statistic mentioned at the top of this article.
I would suggest this value is increasing gradually now because of the cloud native geospatial 2.0 framework as well as the huge amount of data that we are getting access to because of the disruptive upstream space sector with all the next generation satellites. Along with that we have an increasing implementation of an agile solution approach in our project and solution development enabling more collaboration between stakeholders and helping to speed up solution validation.
Dr. Yajnaseni Palchowdhuri, Earth Observation Consultant, Cyient
Data scientists need to work hand in glove with business and IT. MLOps is a collaborative function, often composed of data scientists, devops engineers, and IT. By adopting an MLOps approach, data scientists and machine learning engineers can collaborate and increase the pace of model development and production. Working with business, managers can also help to discover new applications for machine learning that will drive business results.

In Picterra, data labeling is a straightforward and efficient process and as the complexity and volume of your images increase, so will your need for input from colleagues during the labeling process. To improve data quality over time, your model must incorporate business rules, context, and edge cases. Collaborative features within the platform contribute to model accuracy improvement by facilitating label auditing. Anyone within the organization with a Picterra account can log in to verify the accuracy of labels and update them as necessary. It also counteracts the effects of human error or bias of individual annotators.
As well as counteracting human error, geospatial MLOps supports the monitoring of the model accuracy to best improve it. Once the model has been trained within Picterra it can be evaluated using testing and accuracy areas. This model evaluation is an iterative process which when combined with the collaborative features already described enables improvements in the quality of training data and thus more accurate results. The improvement in data quality leads to increased model performance. Business users and data scientists can contribute to ML initiatives using our intuitive platform without sacrificing flexibility and scalability.
Scale from prototype to production faster
Leveraging geospatial MLOps and a software platform such as Picterra also allows for effective & rapid scalability from prototype to production. This means that many more ideas can be explored at a rapid pace, with the ability to scale the great ones more efficiently. This time to market and velocity is key and was outlined by Cyient during the webinar:
Every solution goes through a defined journey, from proof of concept, to prototyping the MVP, and moving into production… the time and effort we spend in the feasibility and efficiency study of the PoC holds the key for production scalability… and we have seen that the time and effort is enormously reduced when we use an automated cloud based platform like Picterra
Dr. Yajnaseni Palchowdhuri, Earth Observation Consultant, Cyient
Dr. Palchowdhuri also went on to describe various use cases where they have been able to move from successful PoCs to production in a very short amount of time where with qualitative and iterative training, detection accuracy jumped from 60 to over 90%. The rest of the interview focused on a few key areas with Dr. Palchowdhuri’s responses paraphrased as follows.
The need for geospatial MLOps
Geospatial machine learning helps us to speed up this analysis, accelerate assessment from earth observation imagery. With all the huge amount of data accessible to us from all these next gen satellites, human based data processing and analytics became impossible to scale up at a regional or national level. With MLOps we can produce ready to use insight within hours. The main need is rooted at the benefit it offers in terms of saving time
Dr. Yajnaseni Palchowdhuri, Earth Observation Consultant, Cyient
Stand out from the competition
The first thing that stands out is how quickly we can perform and implement a successful PoC. Traditional machine learning has several phases of development; requirements, feasibility, processing, calibration and then finding the best fit analytics for a specific use case. In the past this would usually take between 2 to 3 months but by using Picterra we are able to go into production after just 1 month, saving almost 60% of time. Secondly the iterative learning and constant model improvement that we can perform also gives us scope for constant validation and if we are going in the right direction or not. Support and feedback between our own and Picterra’s engineers is regular and transparent. All of this allows us to build scalable and robust data models that can be applicable to any geography.
Dr. Yajnaseni Palchowdhuri, Earth Observation Consultant, Cyient
Drive more value for clients
Customizing an efficient and self-sustaining solution for each use case and client, from planning to designing and deployment, requires a considerable amount of time before showcasing any outcomes to the client. Picterra speeds up this process by providing a baseline template on which each customer solution can be tailored. Quicker testing and iterative learning can improve the model confidence giving the stakeholder more time to validate their need and expectation. Finally, it can also serve as a quick demo interface for potential customers to showcase that the use case is viable. For example we recently ran a quick demo on site selection for 5G planning and were able to produce high quality results in just 2 days.
Dr. Yajnaseni Palchowdhuri, Earth Observation Consultant, Cyient
Gain additional internal value
The fast pace of creating a project will definitely help us to expand our geospatial client base and a sustainable client relationship by our robust cloud agnostic solutions. We are also improving our collaborations and communications internally between different skill sets and teams including ML engineers, data scientists, IT, and GIS analysts.
Dr. Yajnaseni Palchowdhuri, Earth Observation Consultant, Cyient
Various functions in your company can benefit from Picterra in different ways:
Geospatial MLOps is crucial to help machine learning projects make it out of the initial phase. A software platform such as Picterra allows companies like Cyient, to build applications and services at scale with massive amounts of data that are integrated with existing workflows.
Picterra provides a unified geospatial ML platform that greatly reduces the time to market and increases the scalability of geospatial ML operations for customers.
By having all of your data in one self-service platform you can prototype faster, produce more models, and penetrate customer accounts more deeply. Make up for a lack of data science skills by automating and simplifying your workflows and ultimately providing more value to clients.
Want to learn more? Request a demo!