The use of UAV (Unmanned Aerial Vehicle) devices is revolutionizing the world of agriculture. When discussing Precision Agriculture and the use of drones in the report: “An eye in the sky for agriculture: the drone revolution” the Food and Agriculture Organisation writes “In the current milieu, use of sustainable information and communication technology in agriculture is not an option. It is a necessity.”
According to a study by Munich Reinsurance American Inc., almost three in four U.S. farmers are “currently using or considering adopting drone technology to assess, monitor and manage their farms”.
Jason Dunn, Strategic Products Expert from Munich Reinsurance American Inc. said: “Whether a farm has less than 100 or more than 5,000 acres, drones can be the eyes and ears for farmers that want to efficiently and cost-effectively monitor and manage crops, livestock, and soil conditions.”
Case study
A coffee producer
UAVs enable farmers, with new types of data, to monitor their yields. The farmers have more accurate information to make better decisions and focus on what they are good at.
While much of the software used in Precision Agriculture allows analysis of NDVI (Normalized Difference Vegetation Index) of the yield, much more information can be extracted and analyzed from this data. Thanks to our innovative & proprietary use of Machine Learning, Picterra empowers users with the ability to create custom detectors, that identify, count, and localize objects identifiable in drone and satellite images. Agriculture farmers can, for instance, automatically segment their fields or count the number of plants.
The creation of a customized detector allows variable application at different moments:
- Harvest yield estimation by counting individual crops at initial emergence within fields
- Identification and location of disease or loss of yield by comparing two sets of images and selection of a specific results area
- Set up of crop stress mapping with agronomy survey targeting
One of our clients, an arabica grower in Brazil, uses the Picterra platform to detect and monitor his coffee crops. Using a drone, he produced detailed imagery covering 8 hectares of fields,at a high resolution of 5cm. He then created a personalized detector to automatically detect the coffee crops within his fields. In utilizing the detector, he was able to arrive at an estimation of harvest yield, which proved much more accurate than previous manual counting methods, as he knew the exact number and placement of crops. Later in the season, the grower identified the least productive areas of these fields by comparing the number of plants in each area. With such analysis, he was able to focus on zones of the field that needed particular attention. Moreover, he now uses far lower levels of pesticide overall. The final result is an increase in his crop yields by 10%.
So, in less than 10 minutes, this grower has created a detector to count the number of arabica crops within his fields, and in just a few clicks, he obtained the results and analysis of the specific objects targeted by his custom detector.
How you can create a custom detector
Using the Picterra platform, anyone can access and utilize our Machine Learning (ML) models easily, with absolutely no prior knowledge of AI or Data Science. The platform requires no coding skills and you don’t need to be a technical expert. To create an ML model detector, the first step is to teach your custom detector what you see, where the relevant information is, what you are looking for, and also what you are not looking for. Let’s explain how you do it step by step.
Upload images
After having created an account, you need to upload images of your project. It can be uploaded in multiple formats (.tiff,.tif, .png, .jpeg) with a maximum size of 10 GB. A second option with the platform is to upload satellite imagery from Mapbox.
In the case of our coffee producer, the pictures are produced by drones with a resolution of 5cm, in .tif format. These images are then uploaded onto the platform.
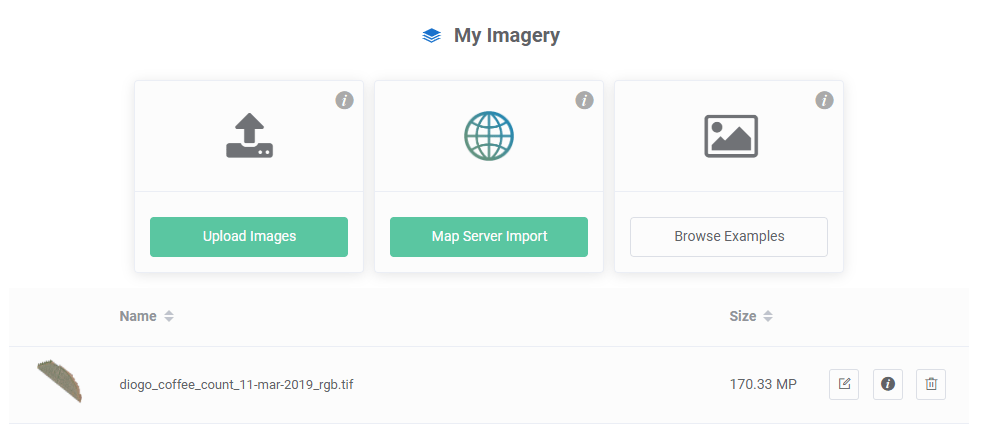
Figure 1: Imagery library of the project
Train the detector
The next step is to create a detector for your use case. Each detector is customized and trained by the user to fit their needs. First, the user indicates to the detector where the relevant information is by drawing training areas. The detector will focus on, and train itself with, information inside these areas. Within the training areas, the user annotates every object that he is looking for, without missing one. The Information which is not annotated inside the training areas is segmentized as non-wanted objects by the detector.
For coffee crop detection, the producer creates a training area with about ten crops. Then, he annotates the crops with circles. In order to improve the accuracy of the results, the producer adds some counterexamples with similar colors. This means highlighting training areas without annotation. It indicates to the detector what the producer is not interested in, such as the forest or the grass.
Iterate the process
Then, the user runs the detector model for a first iteration. The results need to be reviewed to conclude if the detector is performing well. The results will depend on the information given – the training areas and the annotations – and from the diversity of features within the pictures. Thus, the more information you give to the detector, the more accurate it will be. Then, images with lots of different colors, textures & sizes of objects might need more attention and multiple iterations to get accurate results. Consider that if the objects are easy to distinguish by eye, the detector won’t have any difficulty. After each iteration, the detector can be improved by adding more training areas and annotations. Read our article on sheep detection to follow a complete iterative process example.
The case of coffee crops is not complicated and crops are quite easy to distinguish. The first results are already very good. However, to get accurate results on the others, the producer adds a couple of additional annotations of crops and a few more counter-examples.
Get the results
The final results can be downloaded in the format wanted and added to GIS (Geographic Information System) software. It is also possible to generate a .pdf report of the results to include in your project.
The detector is ready to be spread to the entire project and runs on other images. After having invested some time to create a detector, you can deploy it on every image you want with one click, saving you a lot of time.
Want to learn more about custom detector creation, and how it can help your project, save you time and reduce your cost?